In today’s hyper-connected digital landscape, industries like media, broadcast communications, and telecom face unprecedented challenges. Managing complex IT infrastructures while ensuring seamless customer experiences is paramount. Traditional IT operations often fall short, resulting in inefficiencies and consumer dissatisfaction. This is where AIOps (Artificial Intelligence for IT Operations) revolutionises how organisations manage their IT ecosystems.
What is AIOps?
AIOps leverages artificial intelligence (AI) and machine learning (ML) to optimise and automate IT operations. It proactively identifies and resolves issues, improving operational efficiency and customer experiences. By analysing IT data and telemetry, AIOps empowers IT Operations (IT Ops), Development Operations (DevOps), and Site Reliability Engineering (SRE) teams to swiftly detect and address problems, ensuring business continuity and customer satisfaction. According to Gartner, the global AIOps market is projected to reach $8 billion by 2025.
AIOps Platform Components and Workflow
AIOps integrates Big Data, Machine Learning, and IT Service Management (ITSM) to optimise IT operations:
- Big Data: Collects and aggregates real-time and historical data, logging metrics, events, and real user data from applications and the CMDB (Configuration Management Database).
- Machine Learning: Uses advanced algorithms for performance analysis, anomaly detection, correlation, contextualisation, historical analysis, and knowledge management.
- AIOps Platform:
- Observe (Monitoring): Constantly monitors IT environments.
- Engage (ITSM): Facilitates IT Service Management by addressing issues and service requests.
- Act (Automation): Automates responses using scripts, runbooks, change risk analysis, and ChatOps.
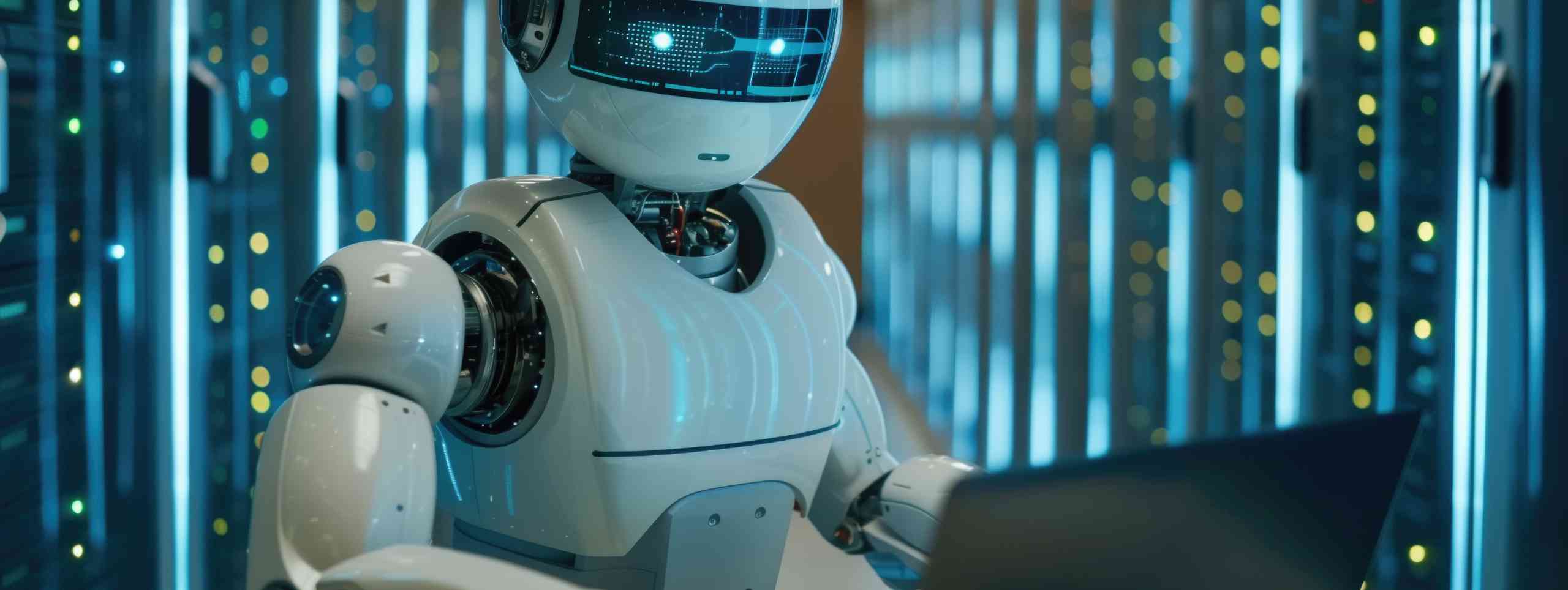
Key Applications of AIOps
Observability
Aggregates and analyses log data, metrics, and traces.
Anomaly Detection
Quickly identifies irregularities, reducing downtime.
Log Analysis
Speeds up mean time to resolution (MTTR).
Event Correlation
Optimises content delivery routes, reducing buffering.
Predictive Analytics
Forecasts network traffic patterns.
Real-Time Alerting
Provides real-time alerts, reducing incident response.
User Experience Monitoring
Continuously monitors user experience.
Integration with ITSM
Automates incident management processes.
Root Cause Analysis
Accurately identifies network issues.
Automated Remediation
Automatically reroutes traffic or scales resources.
Self-Healing Systems
Automatically mitigates security threats.
Underlying Algorithms in AIOps
- Anomaly Detection – Uses statistical methods, clustering techniques, and ML models.
- Correlation Analysis – Identifies relationships using correlation matrices and Bayesian networks.
- Predictive Analytics – Employs regression models, decision trees, and neural networks.
Key Steps in AIOps Implementation
1. Assessment
Evaluate current IT operations and identify pain points.
2. Strategy Development
Define goals and objectives for AIOps implementation.
3. Tool Selection
Choose the right AIOps platform to fit organizational needs.
4. Data Integration
Ensure seamless data integration from various sources.
5. Pilot Deployment:
Test the platform with a pilot project.
6. Full Deployment:
Roll out the platform across the organization.
7. Continuous Improvement:
Monitor performance and make iterative improvements.
Deciphering the AIOps Landscape: Solution Types
1. Domain-Centric AIOps
Focuses on a single domain using dedicated data collection mechanisms.
Examples: Moogsoft, Splunk ITSI, AppDynamics, Dynatrace, New Relic
2. Domain-Agnostic AIOps
Operates across multiple domains, integrating data from varied sources.
Examples: Apache NiFi, Talend, TensorFlow, IBM Watson AIOps, BigPanda
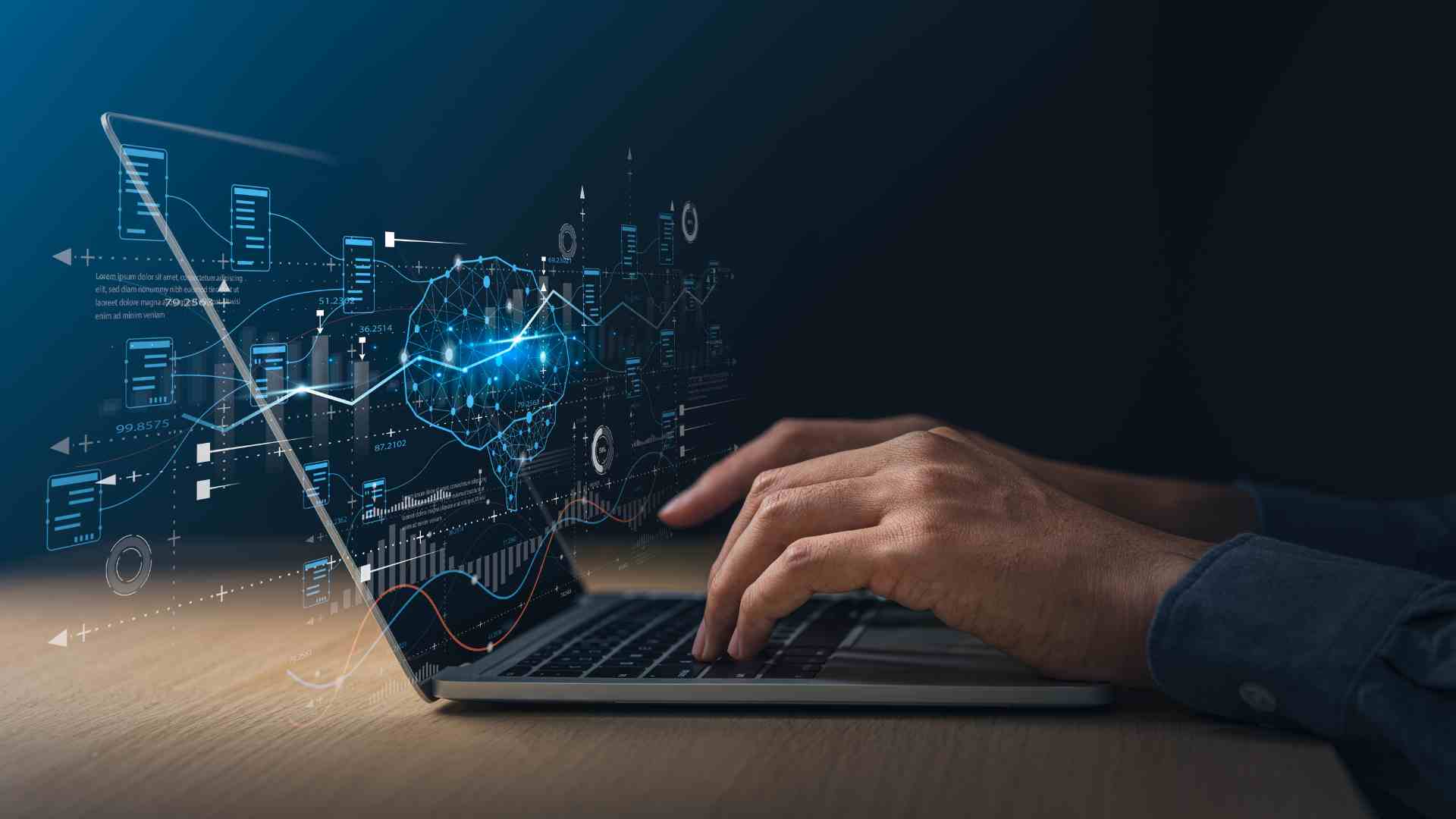
TEDAX: Tata Elxsi's Data Analytics Platform
TEDAX exemplifies an AIOps solution, integrating the following advanced components to revolutionize IT operations:
Data Ingestion and Processing
Handles large data volumes, enabling real-time processing.
Advanced Analytics
Uses ML and AI to identify patterns and trends.
Event Correlation
Reduces MTTR with sophisticated correlation techniques.
Predictive Maintenance
Reduces downtime through predictive analytics.
Automation
Streamlines routine tasks, empowering IT staff.
Visualisation and Reporting
Provides actionable insights via intuitive dashboards.
Scalability
Supports increasing data volumes and analytics needs.
Security and Compliance
Ensures robust security and regulatory compliance.
Overcoming Challenges in AIOps Implementation
1. Data Integration and Quality
Implement robust data management strategies.
2. Skills Gap and Talent Shortage
Invest in training and recruit data science, ML, and AI talent.
3. Tool Sprawl and Complexity
Streamline IT toolchain with comprehensive AIOps platforms.
4. Organizational Resistance
Communicate benefits, provide training, engage executive sponsors.
5. Scalability
Leverage cloud-based solutions and distributed architectures.
6. Change Management
Provide comprehensive training, ongoing support, and early stakeholder involvement.
Best Practices for AIOps
- Start Small, Scale Fast: Begin with pilot projects, then scale up.
- Focus on Data Quality: Ensure data accuracy and relevance.
- Collaborate Across Teams: Align AIOps with business objectives by fostering collaboration.
- Continuous Learning and Improvement: Invest in training to keep pace with AI and ML advancements.
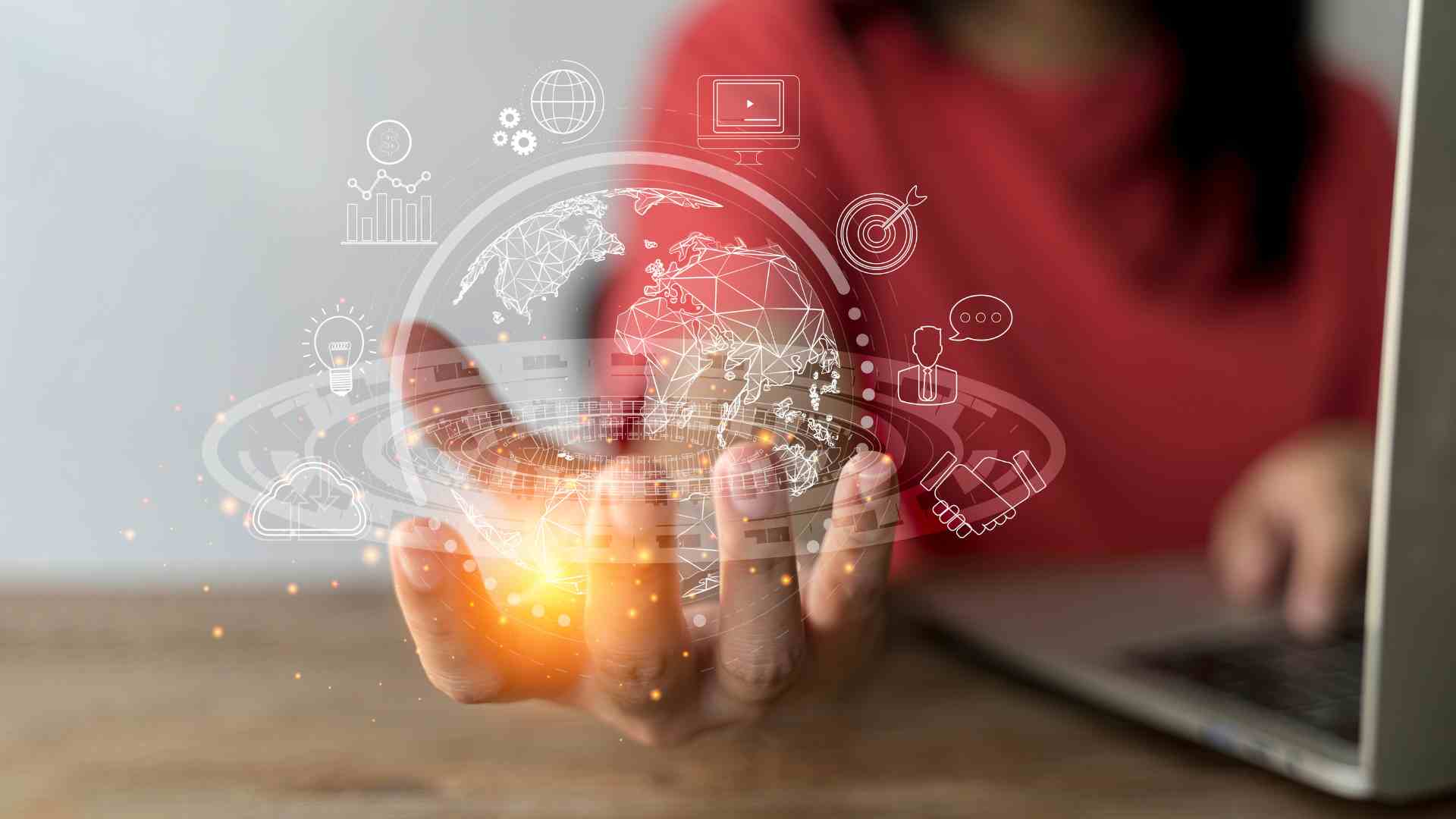
Use Cases and Examples
Network Performance Management
Analysing traffic patterns to optimize performance.
Customer Support Automation
Automating support ticket triage and resolution.
Personalized Content Recommendation
Tailoring recommendations based on user behaviour.
Network Security Enhancement
Real-time threat detection and mitigation.
Quality of Service Optimization
AI-driven analytics for optimal transmission.
Resource Allocation and Capacity Planning
AI-based forecasting for resource demands.
Social Media Monitoring and Sentiment Analysis
Adapting content strategies based on feedback.
Benefits of AIOps
- Improved Operational Efficiency: Reduces manual effort by up to 40%.
- Proactive Issue Resolution: Reduces MTTR by up to 50%.
- Enhanced Customer Experience: Increases satisfaction scores by up to 30%.
- Cost Reduction: Reduces unplanned downtime costs by up to 60%.
- Accelerated Innovation: Reduces time-to-market for new services by up to 40%.
Future Trends in AIOps
- Integration with DevOps: Enhances CI/CD pipelines and accelerates software delivery.
- Edge Computing: Extends to edge devices for real-time insights.
- AI-Driven Security: Enhanced threat detection and response.
- Hyperautomation: Combines AIOps with RPA for end-to-end automation.
- Increased Adoption: Growing recognition of benefits will accelerate AIOps adoption.
As AIOps advances, it intertwines with cutting-edge concepts like Dark NOC, paving the path towards NoOps, where operations are fully automated, eliminating the necessity for dedicated operational teams.
Embracing AIOps and progressing towards NoOps enables organisations to achieve remarkable enhancements in operational efficiency, elevate customer satisfaction, and realize substantial cost savings.
With continuous advancements and accelerating adoption, the horizon of AIOps gleams with promise, poised to steer further innovation and reshape the landscape of IT operations.