At a Glance
- The journey from driver assistance to fully autonomous vehicles is fueled by cutting-edge AI, sensor fusion, and V2X technologies, making “human-like” decision-making a reality.
- Overcoming challenges like safety and regulatory compliance requires “robust validation” and “extensive testing” to ensure trust and reliability.
- Advanced ADAS solutions are “paving the way” for a driverless future, accelerating the transition with innovations that turn visionary concepts into practical, everyday technology.
In the current technological revolution, AI has become crucial, particularly in driving the automotive industry towards autonomy. As noted in a McKinsey report, ADAS and autonomous driving could generate $300-$400 billion in the passenger car market by 2035, bolstered by consumer interest and commercial solutions available today.
AI at the Wheel: Driving the Shift Towards Autonomous Vehicles
From executing rudimentary calculations to orchestrating complex computations and rendering robust decisions, AI has emerged as a pivotal force driving innovation. Its real-time processing of complex statistical data is demonstrated by features like Traffic Jam Pilot and Highway Assist with Auto Lane-Keeping. These functions, combined with multi-sensor processing and hands-free driving capabilities, highlight its versatility and reliability. As a result, AI has seamlessly transitioned from being a mere tool to a transformative solution, accelerating the evolution towards autonomous driving from the current landscape of Level 2+/L3 ADAS systems.
Major automotive companies have attained proficiency in reaching the initial three levels of autonomy, encompassing features such as Adaptive Cruise Control, Automatic Emergency Braking, and 3D surround view systems. Presently, most advanced market vehicles across various price segments incorporate these intricate features offering Level 2+ autonomy.
The Development of Autonomous Features using AI
Consider the following contrasting scenarios, each illustrating a distinct approach to vehicle safety and navigation.
Scenario 1: Minimalistic Approach for Rear Collision Warning System
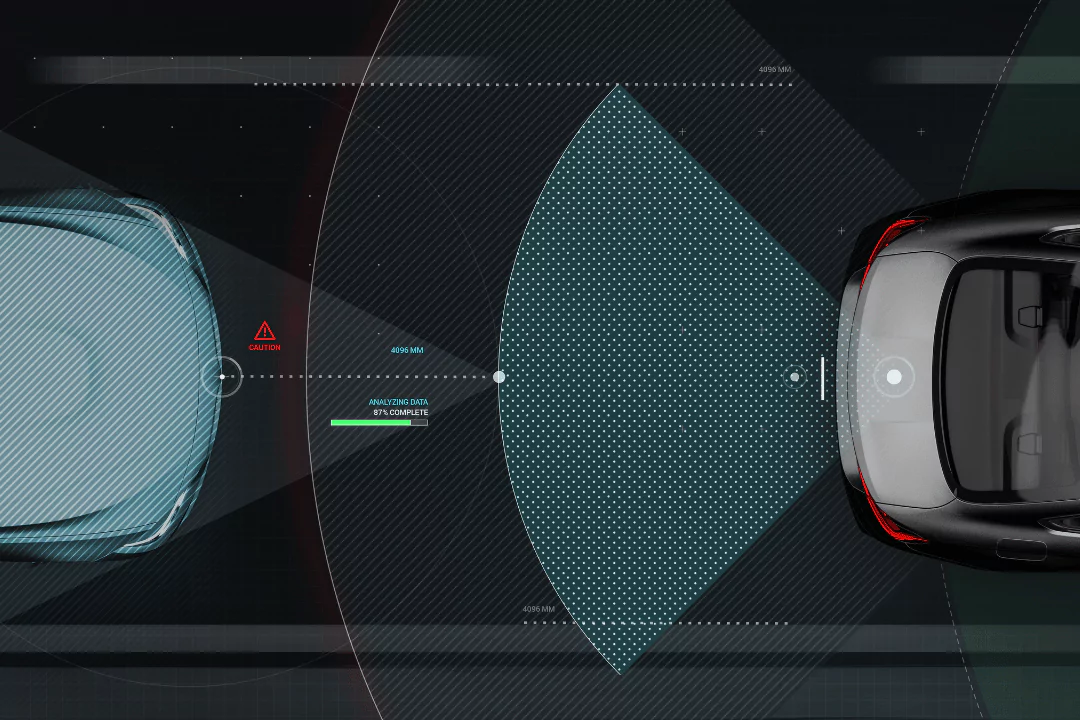
In a quiet suburban setting, a conventional vehicle equipped with a rear collision-warning system approaches a stationary obstacle. The system relies on distance-measuring sensors to detect obstacles and alerts the driver with simple proximity-based warnings. In this scenario, the task involves straightforward data processing, making complex AI and deep learning unnecessary.
A very similar application, Autonomous Emergency Braking (AEB), has been recently implemented by Tata Elxsi in various proof-of-concepts. AEB is a rudimentary requirement for any ADAS solution, which also gives a simple base to design complex systems architectures such as SDV and test them. This system employs computer vision and AI models to measure the distance between the vehicle and objects within its region of interest. Based on collision estimation, the system sends commands to the vehicle’s controller to apply the brakes, ensuring enhanced safety. The AEB solution is tested following the NCAP car-to-car and car-to -pedestrian test scenarios. The Scenarios are tested using the simulator with vehicle running at 30-60 kmph and the traffic is designed according to the NCAP testing protocols.
Scenario 2: Utilising AI for Advanced Path Planning and Navigation
In the bustling streets of New York, a self-driving car equipped with advanced AI and deep learning modules navigates through complex traffic conditions. As it approaches a crowded intersection, the vehicle seamlessly integrates data from various sensors, including cameras and radars, to assess the dynamic environment in real-time. The AI-powered system employs sophisticated algorithms to anticipate the movement of surrounding vehicles and pedestrians, enabling precise path planning to navigate through the maze of traffic with utmost safety and efficiency. By leveraging SLAM (Simultaneous Localisation and Mapping) technology, the vehicle continuously updates its position relative to the surroundings, ensuring accurate navigation even in challenging conditions. In this scenario, AI and deep learning play a pivotal role in orchestrating complex decision-making processes, enabling the autonomous vehicle to navigate seamlessly through intricate urban landscapes.
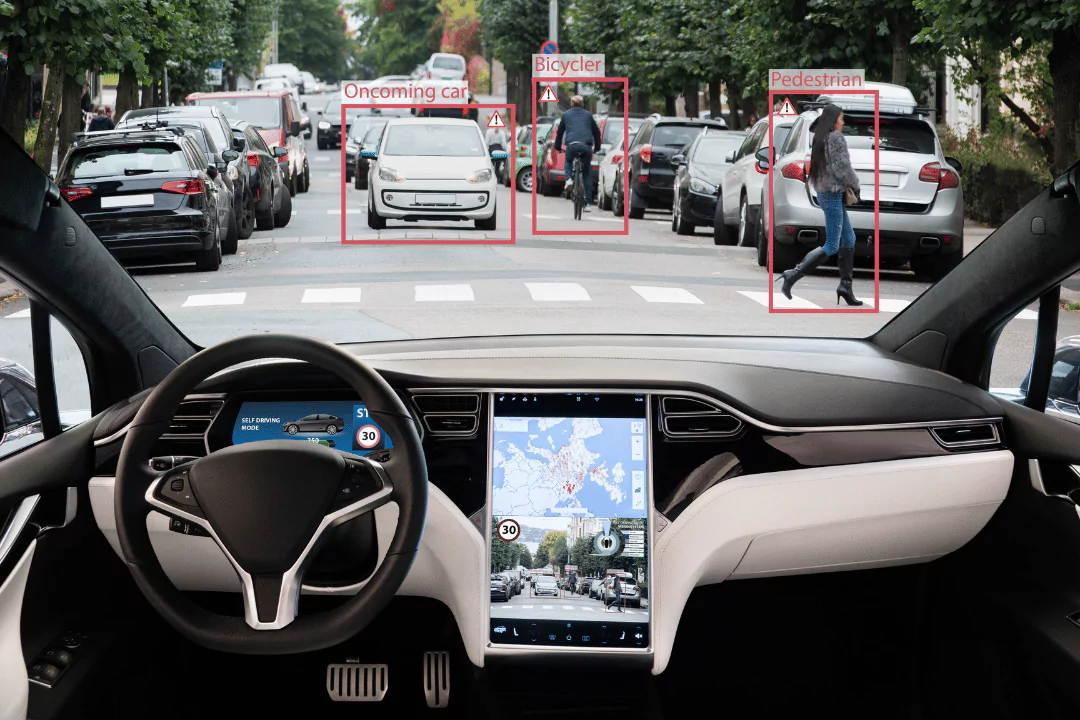
Like the above scenario, Tata Elxsi has developed an auto-parking system, which has been deployed on a Tata test vehicle. One of the challenges addressed is the variation in vehicle trajectory due to different path conditions such as surface level and speed. To estimate vehicle motion at given speeds, the trajectory is recorded. Additionally, real-time processing of GPS, IMU, and vehicle speed data, combined with motion models and path planning algorithms, enables the vehicle to navigate to the target parking position accurately.
Data Requirements, GenAI, and Sensor Fusion
For the Deep Learning models, there exists a substantial demand for datasets encompassing diverse scenarios, tailored to address the nuanced corner cases inherent in devised applications. Gen AI has emerged as a distinctive and expeditious tool for data generation, with a natural learning model embedded at the core of these systems. This inherent adaptability not only facilitates ease of use but also introduces variations within the dataset.
The robustness exhibited in handling highly variable data, renders AI-based solutions advantageous in advancing automation levels in driving. To solve any vehicle state estimation equation relying on such high variable data without any AI implementation causes high computation delay and error in approximation. Furthermore, detecting the obstacles and drivable path is too complex to be resolved by any non-AI based solution. Recently, radars and cameras have emerged as the quintessential sensors for various applications, driving the development of autonomous functions within vehicles. For example, the integration of these sensors—comprising 5 radars, 5 cameras, 12 ultrasonic sensors, and an interior camera and radar—employs different neural networks such as VGG7, Yolo, and CNN. These networks operate in parallel or sequence to make informed decisions.
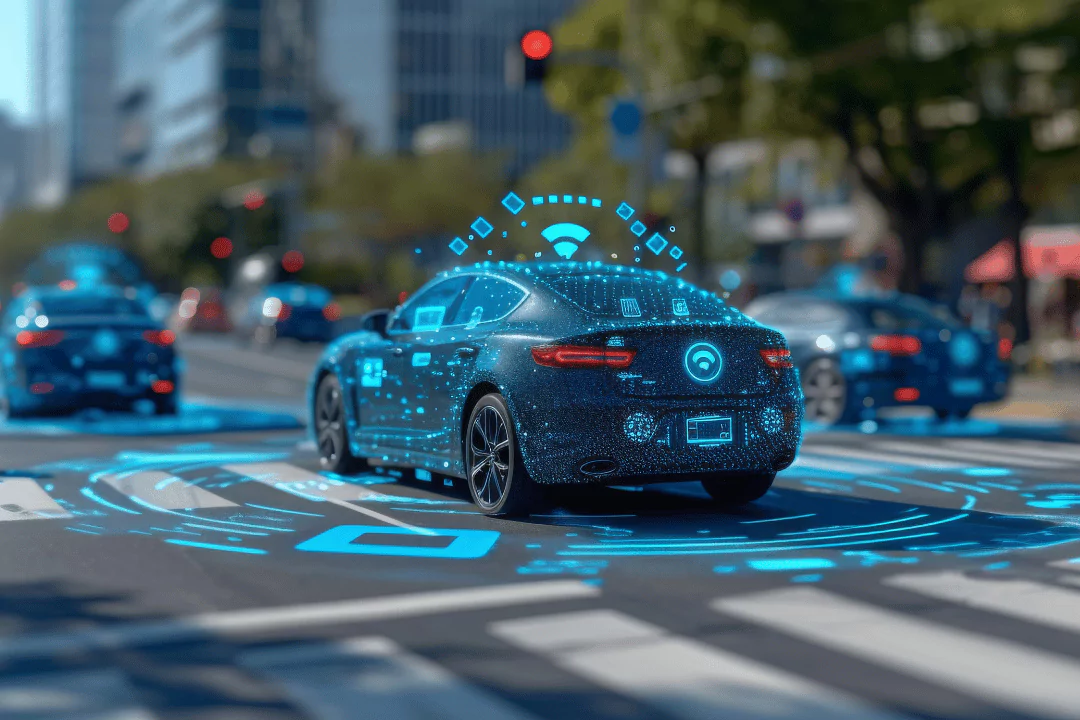
Furthermore, the evolution of hardware accelerators from companies such as Qualcomm and Nvidia, alongside advancements in processing chips, has facilitated the deployment of complex AI models within automotive Electronic Control Units (ECUs). This technological progression underscores the feasibility of integrating heavy AI models into vehicle systems, paving the way for enhanced autonomy and intelligence in driving experiences.
As autonomous vehicles collect and process vast amounts of data via cloud environments, addressing data privacy and the ethical use of information becomes paramount. Ensuring that personal data is protected and that all data handling adheres to stringent ethical standards is crucial for maintaining user trust and complying with legal frameworks in autonomous driving.
Cloud-Based Solutions for Enhanced Vehicle Health Monitoring
The need for increased memory and processing power has catalysed the development of cloud-based solutions. Platforms such as AWS, Azure, and Google Cloud Platform offer a versatile environment for data collection and real-time processing. Leveraging these solutions, AI models can conduct an in-depth analysis of the vehicle’s health status in real time. Beyond health monitoring, cloud-based systems enhance the reliability and safety of sensor data diagnosis, thereby augmenting overall vehicle performance and safety.
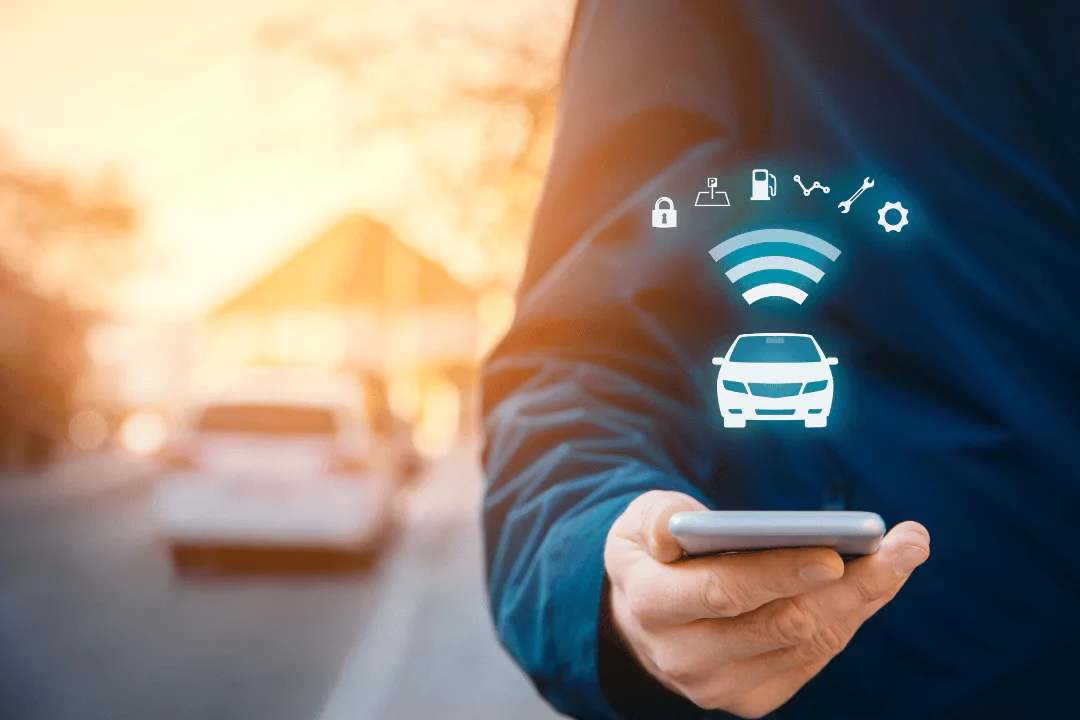
Recently, Tata Elxsi developed a Software Defined Vehicle (SDV) set-up for an ADAS use case as part of a proof-of-concept. This system updates vehicle data, including speed, charge status for electric vehicles, and driver profiles, over the air. The data is transmitted to AWS for performance analysis, battery health monitoring, and driving pattern estimation, enabling comprehensive evaluation and optimisation.
These cloud-based solutions significantly improve operational efficiency in autonomous vehicles which allows for seamless integration of real-time data from various sources, facilitating swift adjustments to driving strategies and maintenance needs. Consequently, vehicles experience fewer downtimes and improved longevity, leading to more efficient fleet management, reduced operational costs, and a delightful customer experience.
TETHER Connected Vehicle Platform for OTA Updates and Fleet Management
Image processing, sensor fusion, system integration, and functional safety compliance.
Use of RADAR, LiDAR, Camera, and IR-based visualisation with optimised AI models and Edge AI hardware
Smart HMI and virtual driver assistance
Robust software and hardware validation services
Realising SDVs with AI
SDV technology revolutionises automotive design by decoupling hardware from software, enabling intensive data processing and computing. The integration of AI and deep learning models within Centralised Vehicle Compute Systems (CVCs) serves as the driving force behind this transformation. These sophisticated algorithms power the command-and-control centre, facilitating real-time decision-making and advanced functionalities. Equipped with FOTA/OTA capabilities, CVCs enable seamless feature updates and new functionalities, leveraging AI to enhance collision avoidance and hands-free driving capabilities. With high performance computing (HPC), SDVs feature scalable architectures capable of accommodating multiple operating systems, enabling the real-time processing of sensor data to enhance safety and user experience.
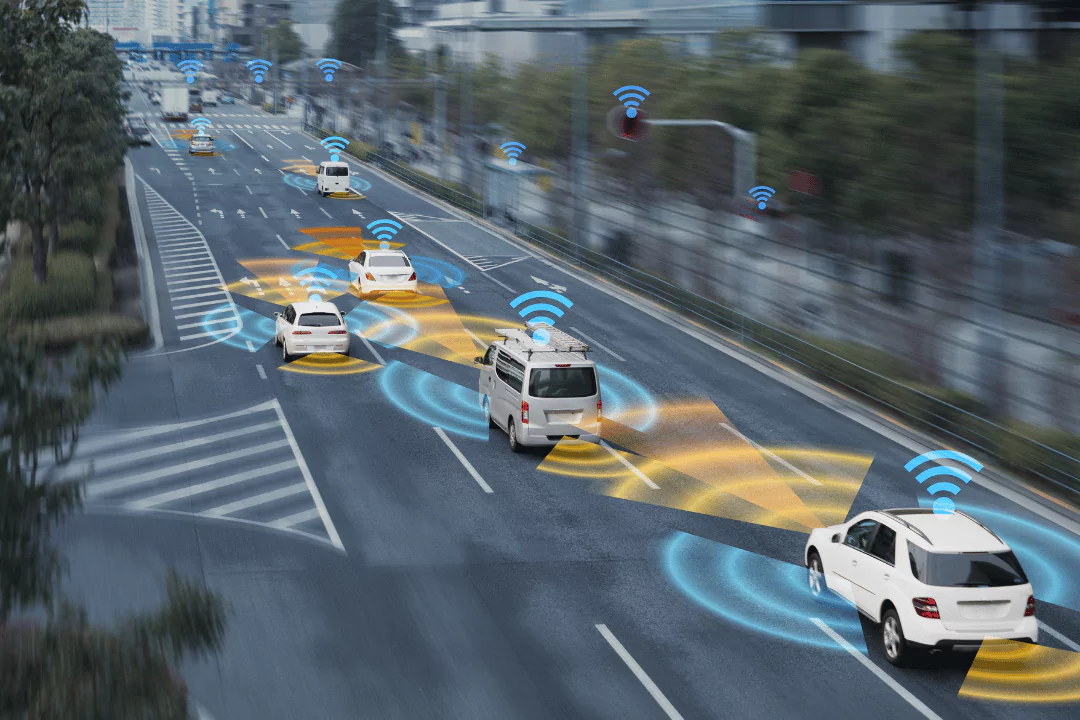
As SDV technology evolves, the integration of AI and deep learning models continues to drive innovation, rendering vehicles safer, more efficient, and seamlessly integrated into our digital lives. Additionally, AI-powered features enable subscription-based access, allowing users to tailor their driving experiences to their preferences, while facilitating the seamless delivery of over-the-air updates, further enhancing the overall driving experience and active safety features in autonomous driving. However, the regulatory and societal implications are profound. As these technologies advance, regulations must adapt to ensure safety and privacy, and society must navigate the ethical considerations of AI in decision-making, which influences public acceptance and trust in autonomous systems.
The Future of AI in ADAS/AD
Looking ahead, the trajectory of AI within Advanced Driver Assistance Systems (ADAS) and Autonomous Driving (AD) promises a paradigm shift towards holistic, streamlined, and real-time interactive driver assistance. With the continued evolution of AI models, the attainment of higher levels of autonomous driving appears imminent. Furthermore, the prospect of personalised driving experiences facilitated by AI assistants tailored to the driver’s profile is on the horizon.
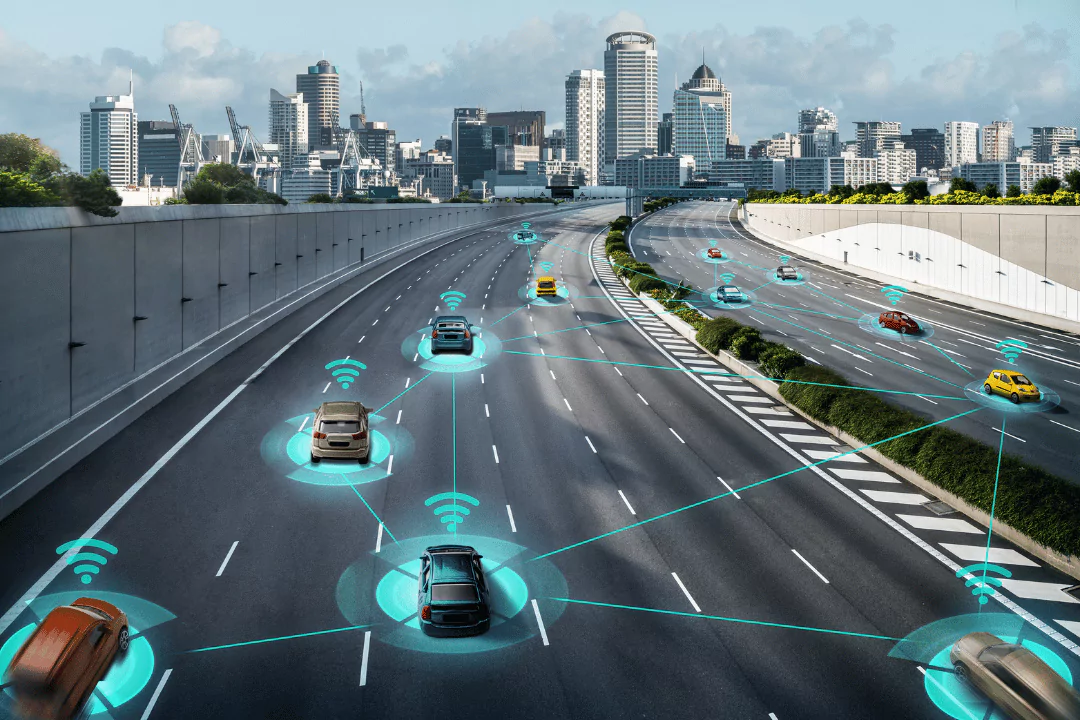
The amalgamation of technological advancements with infrastructure improvements heralds the advent of connected cars in the near future. Enhanced hardware optimisation and swift data transfer are poised to revolutionise Vehicle-to-Vehicle (V2V) and Vehicle-to-Everything (V2X) applications. However, as reliance on data transfer intensifies, potential challenges concerning vehicle safety may arise. The automotive industry remains cognisant of these dynamics and is actively developing safer solutions leveraging the latest technologies.
As we edge closer to fully autonomous driving, how will our daily commutes be transformed in terms of personal productivity and leisure, and what new roles will humans play in an increasingly autonomous driving scenario?
Author
Jagadesh Babu Yallapragada
Practice Head - ADAS-AD and Digital Cockpits, Tata Elxsi
Jagadesh Babu is the Practice Head of ADAS-AD and Digital Cockpits at Tata Elxsi. With over 25 years of experience in automotive product development, software systems, and automation, he has played a crucial role in advancing ADAS business development, execution, and global safety analysis. Jagadesh has led the successful launch of numerous global programs and developed key capabilities in ADAS, Functional Safety, and Cockpit Systems, earning recognition for his leadership and technical excellence.